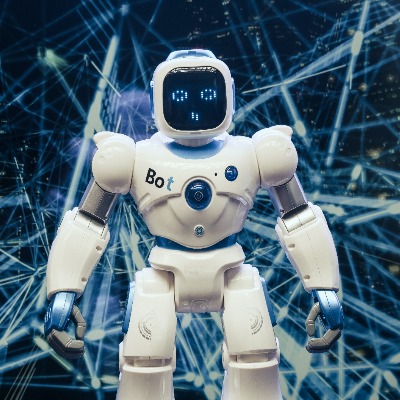
Cancer researchers have many ways to study the biology of cancer and to find and test possible treatments. Examples include growing cancer cells grown in dishes, implanting cancer in animals and even creating three dimensional balls of human tissue known as organoids or tumoroids. However, the accuracy of the findings as they relate to actual human cancer is hard to know. The results from cells in a dish may not be the same as a tumor in a patient.
To help clear the confusion and make cancer research results more reliable, John Hopkins Medicine has developed CancerCellNet, a machine learning tool. They used the tool to examine the behavior of 22 cancer cell lines. A cancer cell line is a group of cells that divide and grow in a petri dish in a laboratory. They are obtained from patient tumors and are used as models of the cancers from which they are taken. The results of the study showed that human cancer cells grown in labs are NOT genetically similar to the tumor types they are supposed to represent. As an example, a cell line called PC3 is used to model prostate cancer, but the study showed that the cells actually behaved more like bladder cancer.
The development of cancer treatments often begins with studies using cell lines. The CancerCellNet results suggest that some researchers may be getting misleading results. Models like CancerCellNet can help to ensure that research results are accurate and reflect what is happening in patients.